Process Modeling & Simulation
High-fidelity LPBF Simulation with Particle Methods
Laser powder bed fusion (LPBF) is the most common additive manufacturing technology for producing complex 3D parts, where a high power density laser is used to melt and fuse powders selectively. This procedure is repeated layer by layer to build or, in other words, "print" 3D structures. Obtaining a fully dense material fabricated by LPBF is a crucial aspect of this production technique that requires optimal deposition and process parameters.
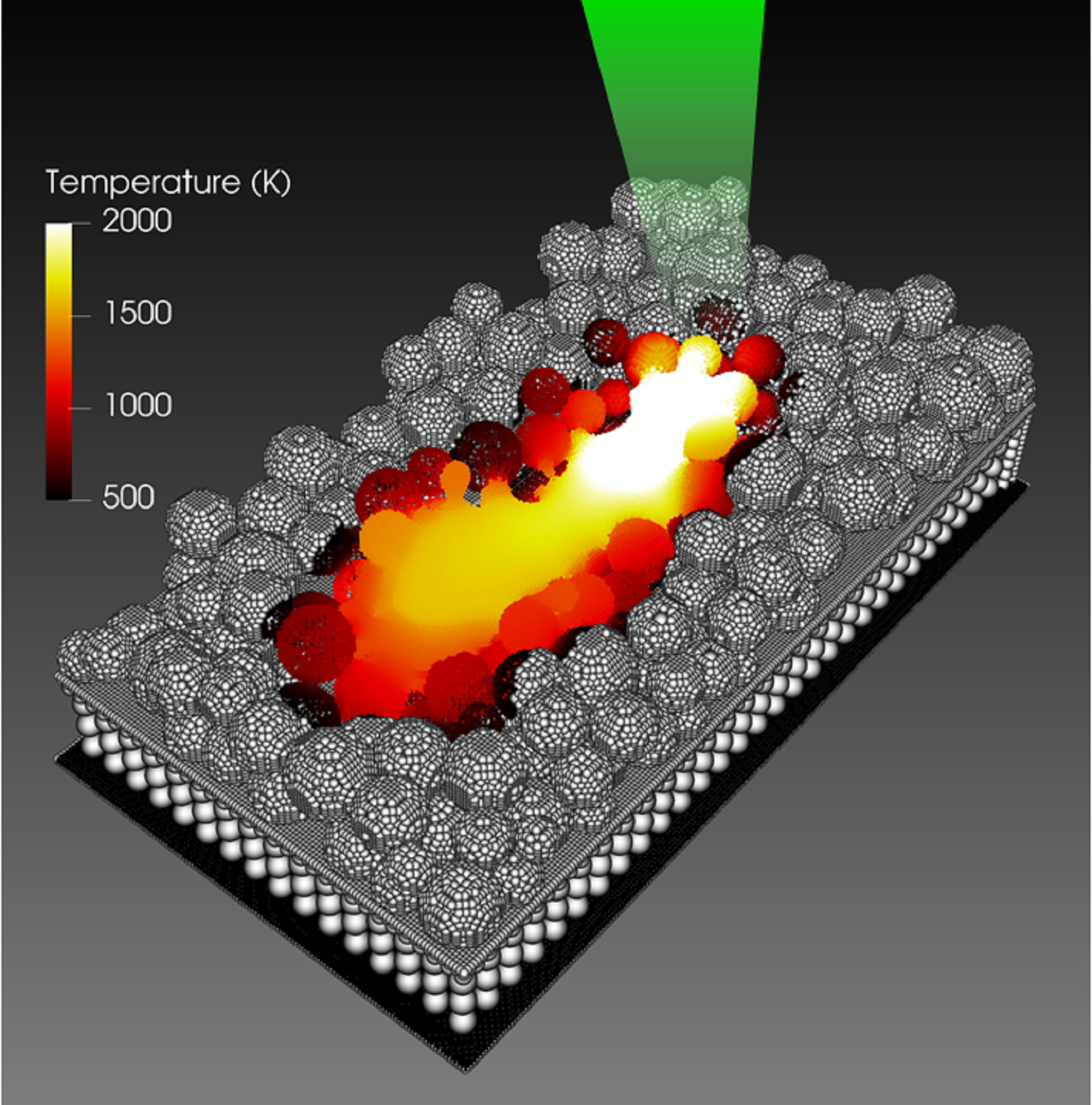
A systematic approach to achieve this goal would fundamentally rely on numerical models as the complexity of various physical phenomena involved in LPBF make the trial-and-error and experimental methods beyond tedious - if possible at all! In this project, a computationally efficient framework based on SPH (smoothed particle hydrodynamics) is developed for modeling and simulation of melt pool behavior during the LPBF process on the powder scale. The main focus will be on minimizing the computational efforts while maximizing the modeling fidelity.
Contact: Christof Lüthi, Dr. Mamzi Afrasiabi
Multiphysics Modeling of Spark Plasma Sintering
Spark Plasma Sintering (SPS) is a modern Additive Manufacturing (AM) technique that can reduce lead times and offer more flexibility in the co-processing of different materials. In SPS, the powders are first compacted and then heated to the point of liquefaction by applying an electrical current. The interplay between the processing conditions and parameters (e.g., pressure, electrical current, heating rate, temperature, and density) is crucial because it defines the final properties of the sintered part. Relying on trial-and-error experiments to understand the detailed mechanism of this process is practically impossible.
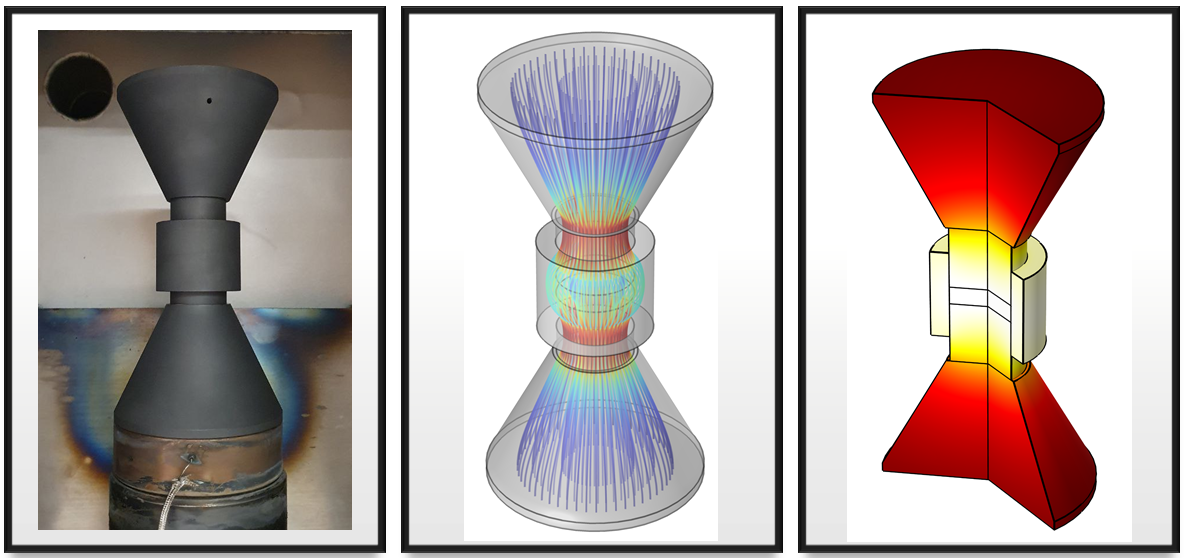
In this project, we strive to develop a multi-physics computational model of SPS for obtaining a detailed description of the process and predicting the thermo-mechanical response of the fabricated part. Commercial FE packages, as well as the in-house particle-based software iMFREE, are utilized for modeling purposes.
Contacts: Ashwani Kumar, Dr. Mamzi Afrasiabi
Inverse Parameter Estimation in Deep Drawing using Automatic Knowledge Acquisition
Deep drawing is one of the most used manufacturing techniques to process metal. The challenges of using this process are monitoring of part quality and controlling the input to provide a robust and consistent final part. This project aims to develop new models and methods to gain and evaluate sensor data about the process. More specifically, we view the observation of part quality as well as other quantities as an ill-posed inverse problem.
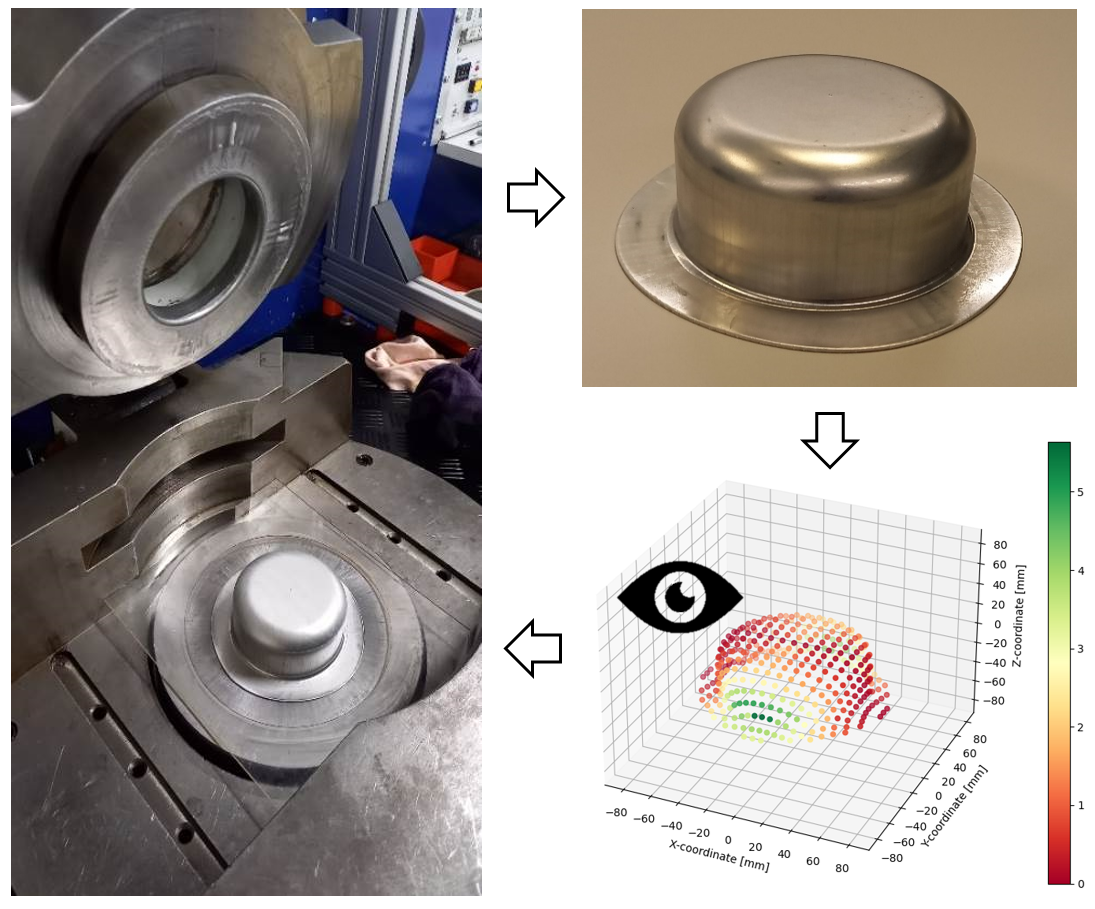
In this problem, we try to find the input and hidden variables which are defined given the process output containing large redundancies, hence ill-posed. In order to solve this inverse problem, we are developing computational methods to find suitable observables, determine mathematically their optimal measurement locations and find predictive models mapping the output to any desired variable.
Contact: Matthias Ryser