Model Predictive Tool-Path Planning

For many complex industrial processes tool-path planning cannot be made first time right. Typically, heuristic planners provide an initial plan that is refined through trial and error during production, which is very time consuming and often no suitable tool-path can be found and serious compromises in part quality have to be made.
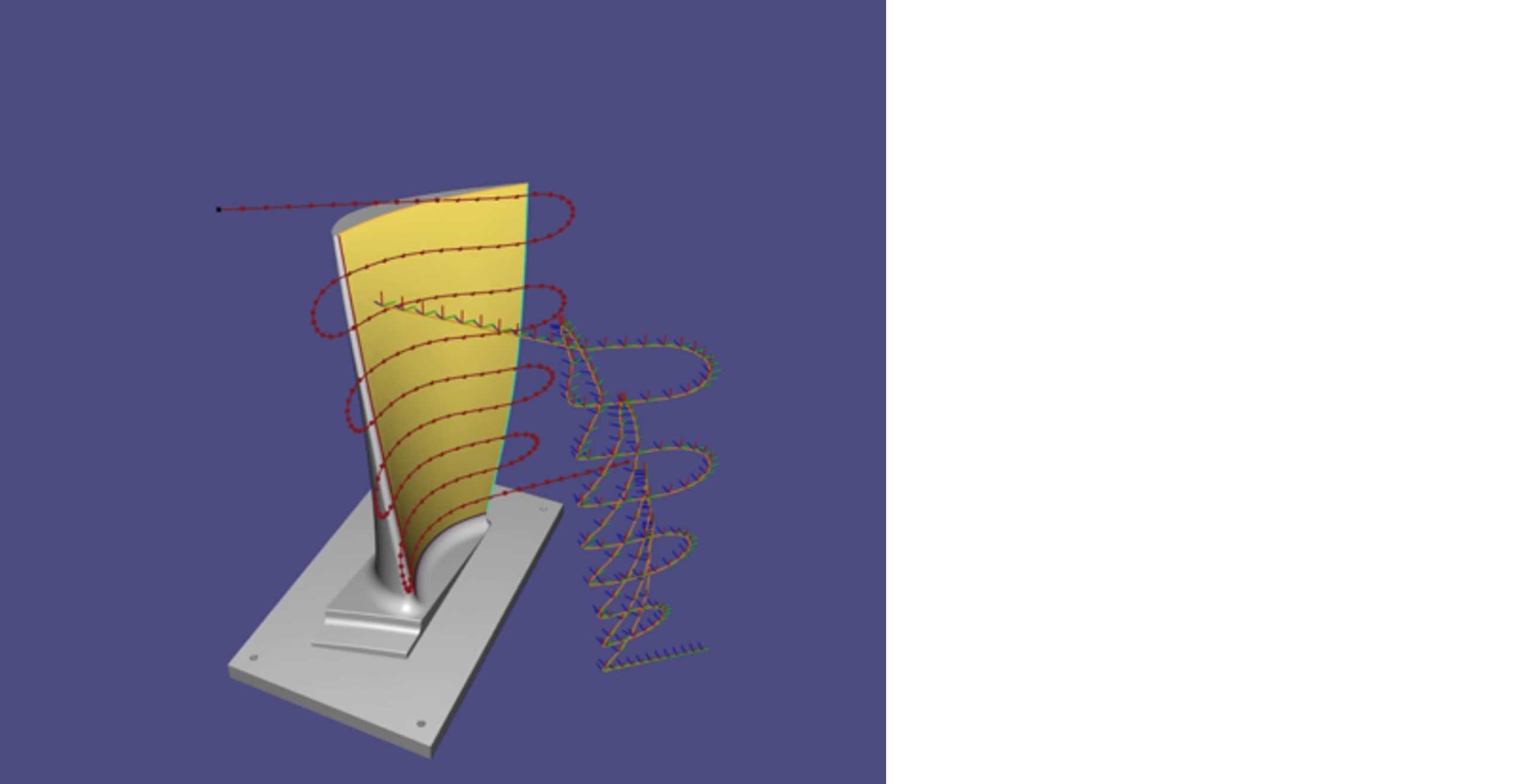
To address this issue, we are developing ultra-fast, data-driven process models that can be automatically calibrated in-line. These models we then use to calculate optimal toolpaths with respect to specific evaluation metrics and setup constraints, utilizing advanced, and most often custom optimization techniques.
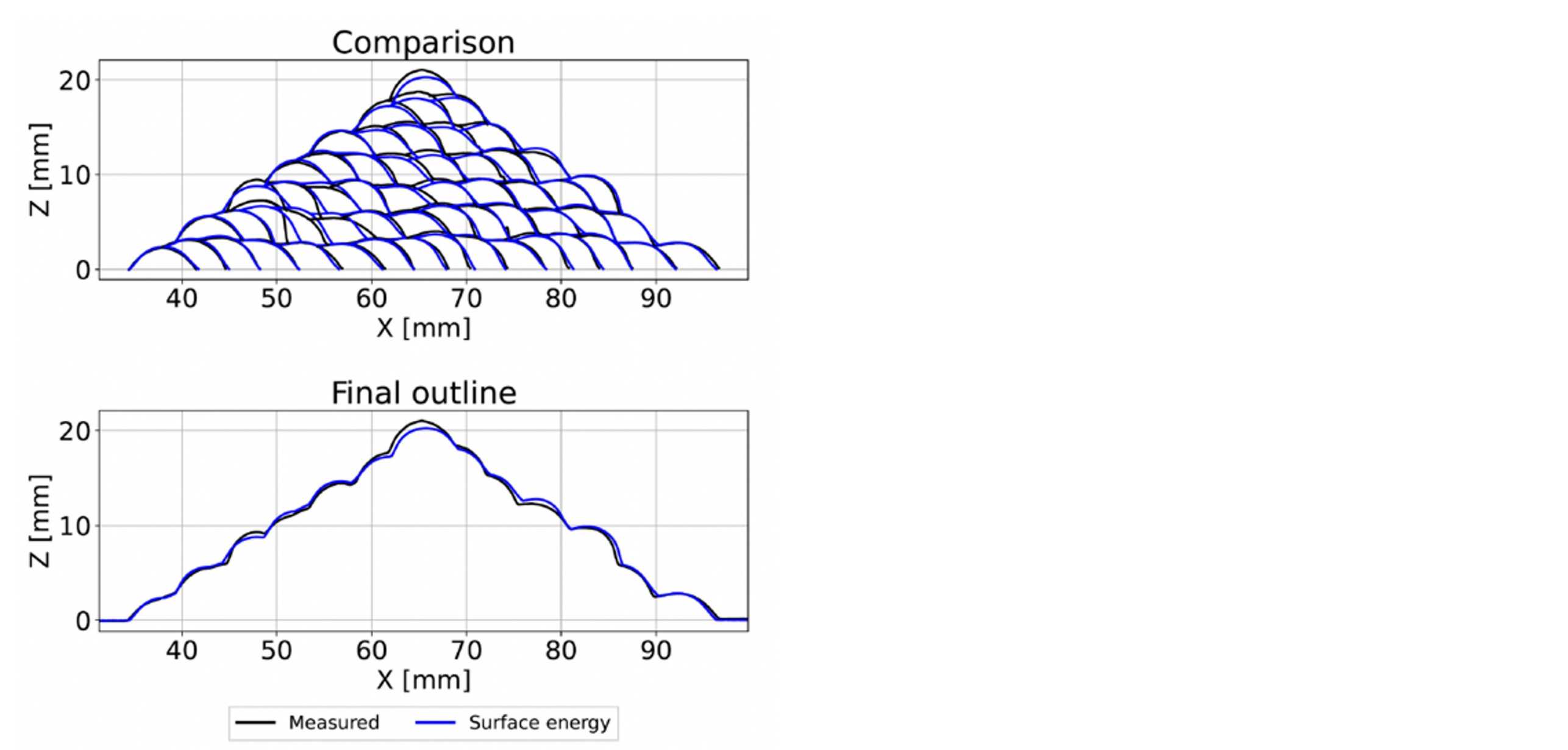
We currently focus on Wire Arc Additive Manufacturing (WAAM) and thermal spraying. In WAAM, we are exploring a reinforcement learning-based planning approach that leverages uncertainty predictions our model provides. In thermal spraying, we are applying nonlinear optimization methods to optimize toolpaths, benefiting from the differentiability of the developed model. In the future, we plan to extend our work to other processes and employ machine learning techniques for generating initial paths, aiming to enable fully automated toolpath generation directly from CAD designs.
For more information about the IRC visit the group-page: www.irz.ethz.ch